The capacity of intermittent power sources like wind and PV is steadily increasing. The existing balance between production and consumption is seriously affected by these new sources. Flexible demand for example in buildings is one solution to come back to a stable system. Buildings provide a huge potential for flexible electricity demand. This flexibility can be addressed by using model predictive control (MPC) with optimized scheduling for the buildings’ heating, ventilation and air conditioning (HVAC) systems. Therefore, it is necessary to have detailed simulation models of each single building. To avoid the huge effort of setting up lots of different building models, two approaches for self-adapting building models are discussed in this paper. Those approaches can be differentiated by their mathematical structure. The neural network (NN) approach is called “black-box” model. In contrast to that, the physical “white-box” model is a system of differential equations with free parameters. These models are parameterized by measured data and are developed to be used in model predictive control to forecast the building’s thermal behavior. Once the thermal behavior is predictable, the optimal schedule at minimal costs for the HVAC systems can be determined with respect to thermal comfort.
The investigations show, that the difference between the training phase and the prediction phase is decisive for the quality of the forecast. If test and training data are similar (e.g. same season), both models deliver satisfying results.
Self-adapting building models for model predictive control
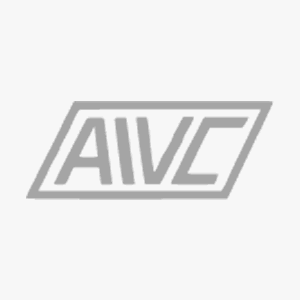
Year:
2013
Bibliographic info:
Building Simulation, 2013, Chambéry, France