Some airborne pathogens can infect susceptible people over long distances in buildings when they are transported in small respiratory particles suspended in the air. The pathogen concentration in air can be decreased using engineering controls, such as ventilation, filtration, or inactivation. To determine their effect, it is common to use the Wells-Riley model to estimate the probability that a susceptible person is infected and is a function of the dose of infectious pathogen received and a Poisson distribution. Wells proposed a hypothetical dose unit, known as the quantum of infection, which is a function of the pathogen emission rate and, in turn, a function of the number of infected people and their individual pathogen emission rates. The quanta generation rate can be determined from the epidemiological data for an outbreak case of a disease in a space where the proportion of a population of people infected with a disease who were initially free of it is known. The quanta generation rate is a temporally and activity varying parameter and so this approach only represents its value at the time the infections occurred and for that space. It is also unique for every disease and disease variant, and the emission rate varies in different spaces because the probability of the presence of infected people also varies. It is unknown at the start of a pandemic, and again later when the pathogen mutation period is greater than the time taken to determine uncertainty in its value. These factors make uncertainty in its value significant and it may vary by several orders of magnitude. A Monte Carlo analysis is used to show that uncertainty in the quanta emission rate for SARS-CoV-2 varies over around 8 orders of magnitude. There is a general paucity of data of sufficient quality to reduce uncertainty in emission rates. This means that there is little confidence in the data located in the tails. The problem with this is demonstrated by applying the emission rates to the WR model to estimate that, for an 8 hour exposure in a 50 person office with an outdoor airflow rate of 10 l s-1 per person, the probability of infection of each occupant from long range transmission is <1% for 95% of events. It is the data in the right-tail of the emission rate distribution that leads to an appreciable probability of infection. These are just a few of several factors that make a probability of infection estimated by the Wells-Riley model unusable as a metric.
Can the Wells-Riley model universally assess airborne pathogen infection risk?
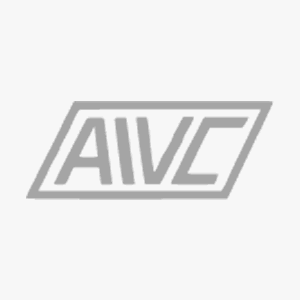
Year:
2023
Languages: English | Pages: 9 pp
Bibliographic info:
43rd AIVC - 11th TightVent - 9th venticool Conference - Copenhagen, Denmark - 4-5 October 2023